How to Make Data Ready for AI
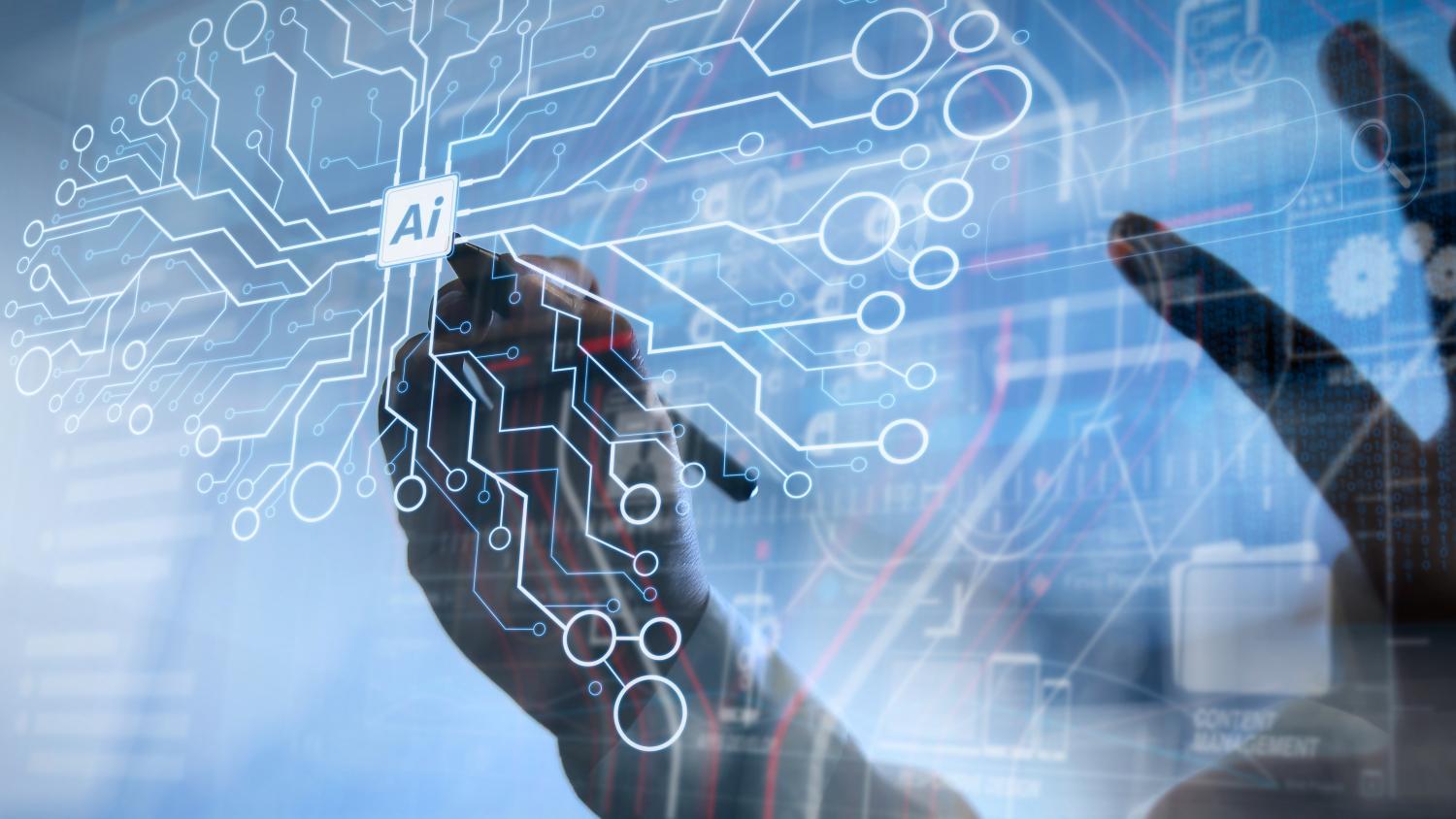
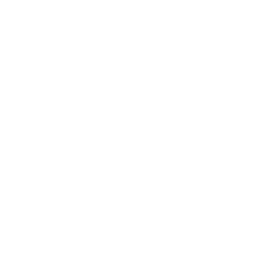
Over the years, I’ve learned that AI may be present in organizations both large and small.
However, regardless of the enterprise’s size, those embarking on their AI Journey may need some help. They’re lacking the experience and equipment from a data perspective.
While AI and machine learning seem universal, how to properly manage the data is a conversation that slips through the cracks.
We must imagine our data in different departments. We need to think of it as siloes, residing in different platforms and repositories.
Detailed + Organized = Efficient.
How Can I Make My Organization’s Data AI-Ready?
The Problem
Companies want to jump directly into the “Fourth Industrial Revolution.” They want to prove that AI can deliver ROI in their businesses.
In reality, companies are just NOW becoming AI-ready, with practical AI still in its early stages. For many of them, it’s the internal company data that’s holding them back.
Many leaders know they have to prepare their data in order to train a model. But how are they to go about it?
This is the difficult part.
The Solution
Creating a unified data warehouse is the goal. This is a central area in your operation where all company data flows in and out and is stored.
Within this data center, your database is cleansed, updated, consolidated, and labeled in a uniform way across every department. This provides a precise baseline for all training data going forward that can be used for any machine learning model you test and deploy.
A unified data warehouse will help make your company data accessible to all, and is necessary to begin creating and personalizing a tool that will work for your business.
Internal Proof of Concept
Once the data is aligned, test the strength (efficacy) of your models with an internal Proof of Concept (POC).
The point of a POC is to prove the possibility of finding business efficiencies. These include things like saving money or improving a customer experience using AI.
This is not an attempt to get the model to the level of accuracy needed to deploy it. It is simply to show that the project can work for the business.
Testing, Testing, Testing
Now it’s time to see what works and what doesn’t. To test, you can do any (or all) of the following:
• Use off-the-shelf algorithms.
• Utilize open source training data.
• Purchase a sample data set.
• Create your own algorithm with internal staff.
Find what works for you to prove that the project will achieve the desired goal.
Ultimately, a successful POC is the baseline to get the rest of the project launched.
Watch Out for Bottlenecks
Training data can be difficult.
Depending on what project you choose, it can take tens of thousands of records to train your model. High-quality data is paramount to project success.
It’s understandable that data science teams often underestimate the quantity of training data they will need. As a leadership team, it’s important to choose initial AI projects where you can pull sufficient amounts of quality data to train your models.
While not enough training data is one common roadblock, it is also essential that you are watching for and eliminating any bias in your data as you go along.
How DataForce Can Help
Your team will want to implement process practices to make adjustments on the fly. This is where the method in which you collect and annotate your data sets is of utmost importance. Bringing on an experienced team to conduct this data preparation can make all the difference in successful AI deployments.
As with anything, you will need to invest time and money here. But with persistence and patience, running a few successful AI tests will help your business and your team exponentially.
DataForce offers AI solutions across multiple industries. Contact us today to learn how we can help.
By Brad Hastedt,
Director of AI Data Solutions,
DataForce