Text Annotation, Search Relevance Rating, Conversational AI Localization, Language Engineering
Query & Response Evaluation and Ranking for Generative AI Chatbot
The Challenge
Our client, an international technology company, was looking for a partner to assist in training its AI-enabled large language model (LLM) in both the relevance and accuracy of prompt query and response interactions. The project had a variety of goals, including:
- Query Evaluation: confirming the query is answerable.
- Response Evaluation: confirming the response is correct, understandable, and complete.
- Response Ranking: confirming the response is both natural and relevant based on the query.
In order to accomplish this, our client needed a partner with the ability to train the model with a variety of queries, paired with multiple responses based on our client’s requirements, all while sourcing a group of qualified contributors who could accurately analyze the data in detail and then categorize and rank it accordingly.
• • • •The Solution• • • •
After understanding our client’s specific requirements, the DataForce team began building an offshore team, leveraging our global network that had the flexibility to add contributors as the project continued to grow. Prior to onboarding, applicants were screened to ensure they could review and answer prompts from the perspective of someone living in the United States.
Once applicants were approved and onboarded, they were given detailed instructions and training material on how to evaluate and rank queries and responses from the chatbot.
Building an evaluation and ranking process with quality assurance in mind:
- Each query and response was evaluated and ranked twice in efforts to find agreement and yield the highest-quality data.
- If the annotators had opposing opinions, a third annotator was brought in to be the tie-breaker.
With this approach set as a standard in the early stages of the project, our client was able to leverage much-needed insights as the annotation team uncovered what did and did not make sense in the variety of queries and responses. For example, an answer could make sense but not be specific and measurable. This real-time feedback from the disagreement rate would reveal that the posed question is too difficult to get into an agreeable state. As the project continued, our client was able to refine project requirements by leveraging the opinions and findings from the annotation team.
Following the initial pilot, our client was very pleased with the progression of the model training and added multiple additional batches of data to be evaluated and ranked. Our client was able to constantly change project requirements based on the real-time feedback all while meeting the agreed-upon timeline.
DataForce was able to provide responses with a high-quality output so our client could utilize the data collected as a valuable asset in training its generative AI chatbot model.
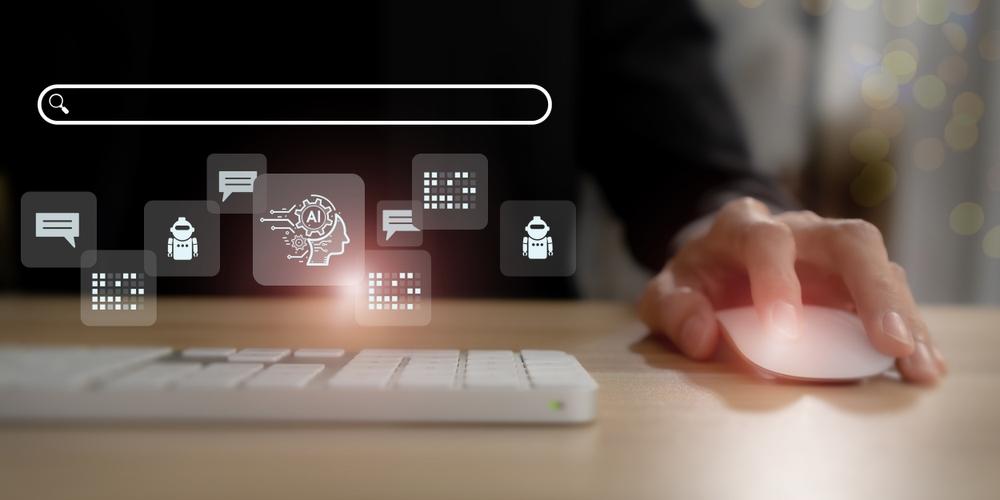
DataForce has a global community of over 1,000,000 members from around the globe and linguistic experts in over 250 languages. DataForce is its own platform but can also use client or third-party tools. This way, your data is always under control.