Building Better AI: Best Practices for Generative AI Quality Rating
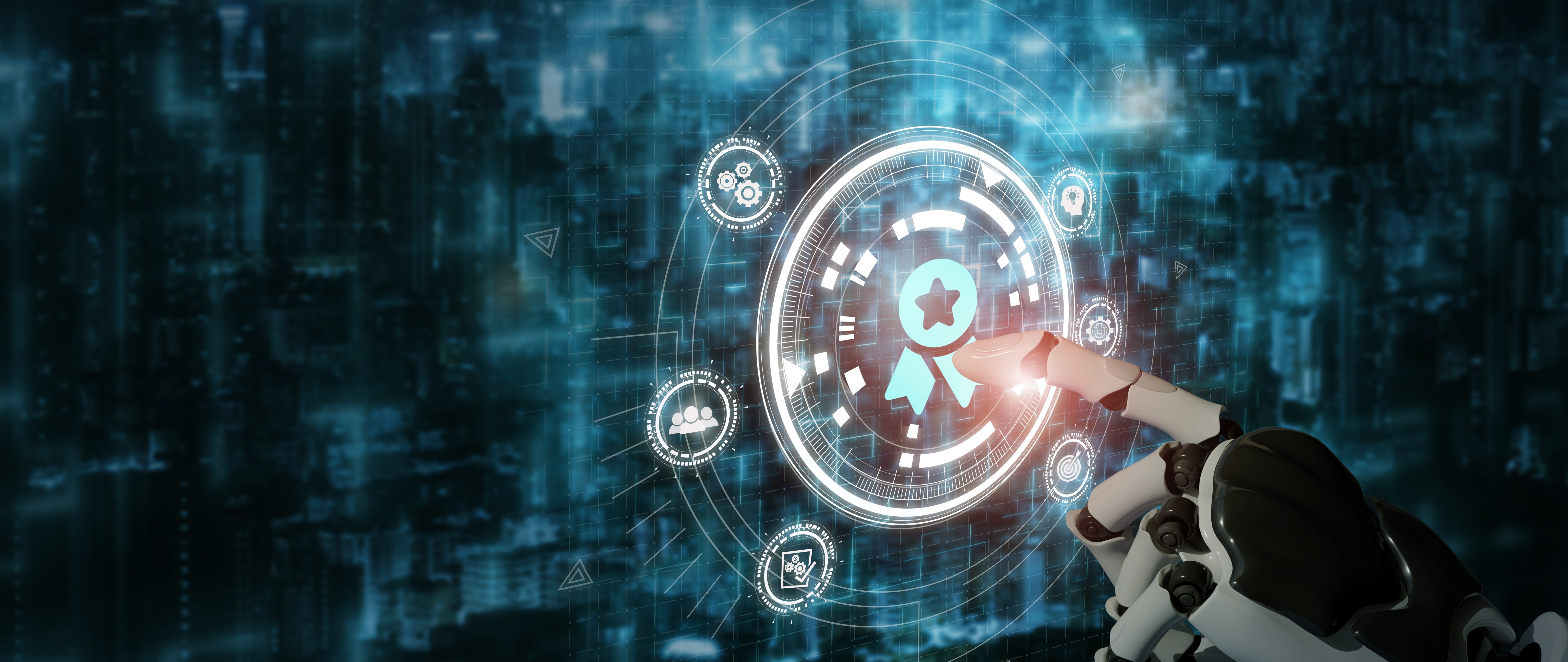
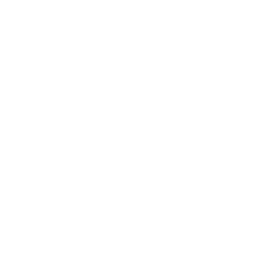
As enterprises increasingly integrate generative AI into their operations, maintaining content quality is a growing priority. From chatbots and virtual assistants to code completion, organizations rely on this powerful technology to produce relevant, accurate, and useful outputs. However, evaluating the quality of AI-generated content at scale is no small feat—traditional assessment methods often fall short. To keep pace, companies must adopt evaluation practices that ensure consistency and reliability at any production volume.
Defining Quality in Generative AI
Generative AI quality extends beyond simple accuracy. Multiple factors must be assessed, including:
- Relevance: Does the output align with user intent or prompt requirements?
- Accuracy: Does the AI generate verifiable and correct information?
- Coherence: Is the output logical and grammatically sound?
- Diversity: Does the AI produce varied and non-repetitive results?
- Bias Mitigation: Does the AI generate fair responses?
- User Satisfaction: Does the output meet the expectations of end users?
Guaranteeing high-quality AI-generated content requires a structured approach that accounts for all these factors.
Best Practices for Scaling Generative AI Quality Evaluation
Implement a Multi-Tier Quality Assessment Framework
A robust evaluation process should include multiple levels of assessment. Automated scoring tools can analyze coherence, grammar, and factual consistency, while human-in-the-loop review ensures nuanced feedback on accuracy and contextual relevance. Continuous user feedback further refines AI models based on real-world interactions and ratings.
See how a structured, multi-tiered assessment approach improved AI chatbot responses in this case study on query and response evaluation and ranking.
Combine Automation with Human Oversight
While automated tools efficiently analyze AI-generated outputs for structural integrity and sentiment, human oversight remains essential for detecting bias, assessing creativity, and reinforcing cultural sensitivity. A hybrid system of automation and human evaluation maximizes efficiency while maintaining quality.
Learn how we successfully blended automation and human review to enhance an e-commerce platform in this case study.
Develop Domain-Specific Quality Metrics
Evaluation criteria for generative AI vary by industry. For instance, legal and financial AI prioritize factual accuracy and compliance, while marketing AI emphasizes creativity and engagement. In healthcare AI, clinical accuracy and regulatory adherence are paramount. Establishing domain-specific benchmarks helps enterprises effectively measure AI performance.
See how domain-specific quality metrics improved product classification and consumer safety in this case study.
Establish a Scalable Rating System
A structured rating system brings consistency to AI evaluations. Standardized criteria for coherence, accuracy, bias, and diversity ensure reliable assessments across both human and automated reviews—fostering greater transparency and trust in AI-driven outcomes.
Explore how we built a scalable rating and classification system to curate safe and unsafe prompts for IBM's Granite Guardian 3.0 in this case study.
Continuously Train AI Models with High-Quality Feedback
AI models improve over time when trained with high-quality, annotated data. Establishing a continuous feedback loop—collecting real-world insights, analyzing errors to identify patterns, retraining models using curated datasets, and redefining benchmarks ensures ongoing model improvement.
Discover how a large-scale data validation and feedback loop enhanced AI model accuracy in this case study on online data rating and validation.
The Path to AI Excellence
Maintaining high standards in generative AI requires a strategic balance of automation, human oversight, and continuous refinement. By implementing structured frameworks, defining domain-specific quality metrics, and combining AI-driven analysis with human expertise, organizations can ensure their AI-generated content remains reliable, accurate, and valuable. At DataForce, our quality rating services and generative AI solutions empower teams to enhance AI performance through human expertise and advanced AI training. Contact us today to learn how we can support your AI models.
By DataForce