Named Entity Extraction
Adverse Event Detection
Using Natural Language Processing for Adverse Event Detection.
The Problem
Our client, a leading pharmaceutical company, is developing an NLP algorithm for adverse event (AE) detection in social media entries. They aim to categorize pre-defined named entities in order to organize and address specific patient concerns and experiences. More than ten thousand social media entries needed to be analyzed to improve their patient experience and health, as well as reduce the number of false negatives in AE detection. Due to the sensitive medical nature of the project, our overall expectation was to reach 100% accuracy for all categorizations and detections. This human-in-the-loop approach increases scalability by 10 times as, unlike current practices, human review is only required where the machine learning model fails to provide a confident classification result.
• • • •The Solution• • • •
The project started with an efficient selection of subject matter experts from DataForce’s community of one million people. We built the team gradually from screened candidates, handpicking the reviewers who had a background in pharmacovigilance. A combination of expert supervision, a blind annotation process, and the implementation of specific quality metrics such as Kohen’s Kappa coefficient resulted in quick quality improvements. As the annotation process continued, we scaled up the annotation team to shorten the deliverycycle. Our team reviewed thousands of social media posts in a few days.
To guarantee accuracy in annotation and categorization, we performed double-blind annotation on each task, followed by thorough quality controls. Any disagreements were reconciled by a reviewer who came up with the final categorization for each entry.
We implemented feedback promptly with the team as more data sets were coming in, and updated guidelines to reflect edge cases and unexpected experiences within social media posts. Our internal annotation platform, DataForce, updated seamlessly to adapt to all project changes and allowed us to complete the dataset with a scalable model of constant improvement.
The client reported that this human-in-the-loop model proved to be a much more scalable and efficient option than traditional manual categorization.
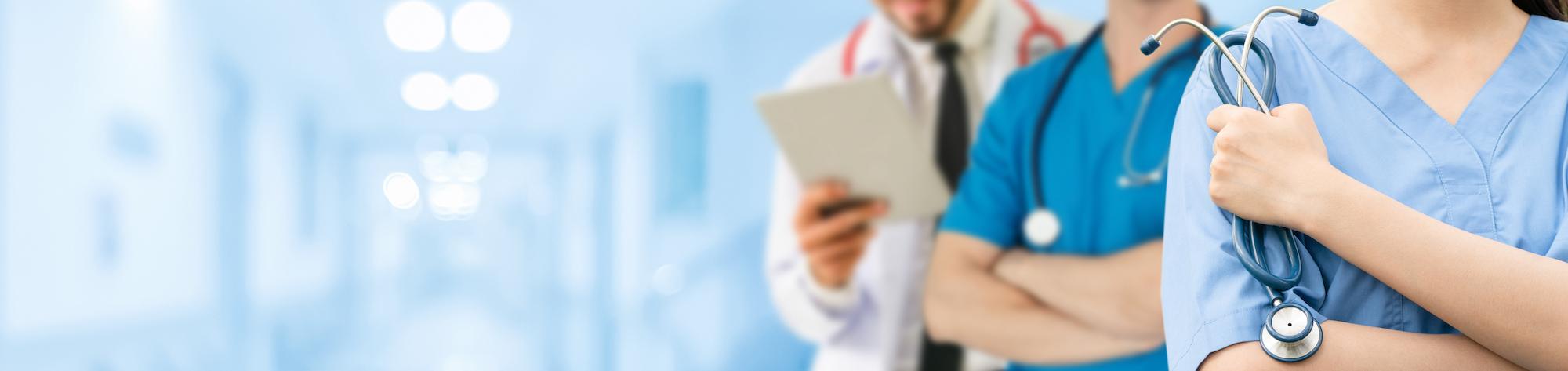
DataForce has a global community of over 1,000,000 members from around the globe and linguistic experts in over 250 languages. DataForce is its own platform but can also use client or third-party tools. This way, your data is always under control.